Technology is becoming more and more integral to our daily lives. Artificial intelligence (AI) in our home is increasing, for example, through personal devices – things like smart speakers, streaming service recommendations, and digital ads on our browsers and in social media feeds.
Duke Law Professor Arti Rai, JD, Examines Challenge of Secret Data Associated with AI in Medical Care
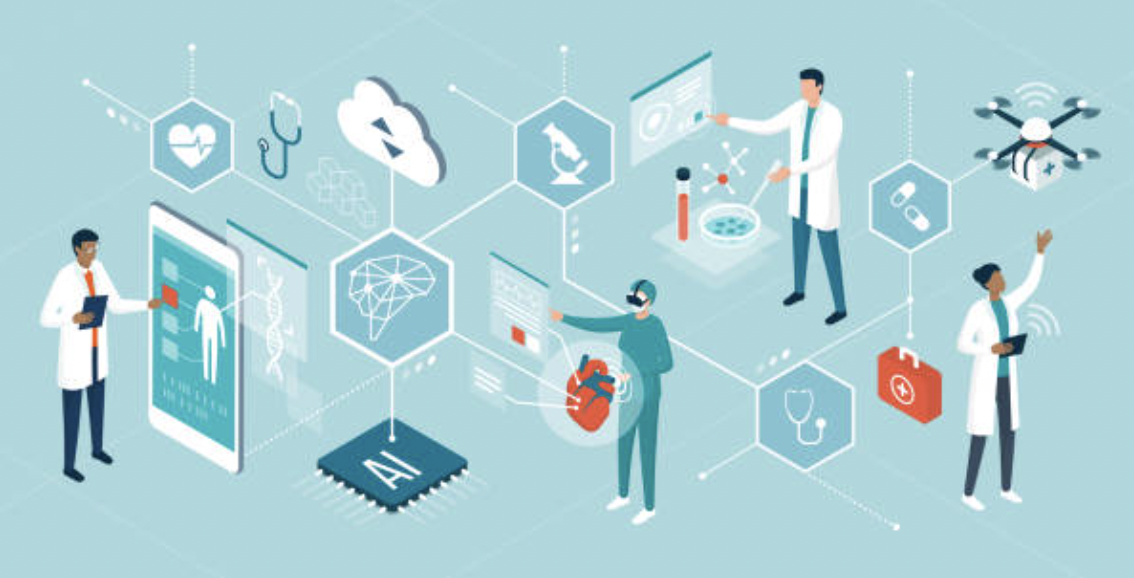
AI is also playing a bigger role in medical care. For example, developments in medical AI known as “clinical decision software enabled by machine learning” (ML-CD), have raised questions of intellectual property law and regulatory requirements. In 2021 the U.S. Food & Drug Administration (FDA), a key regulator for medical device software, stated that “[AI] and machine learning (ML) technologies have the potential to transform health care by deriving new and important insights from the vast amount of data generated during the delivery of health care every day.”
But how does FDA regulation account for the level of secrecy that comes with private product developers’ understandable desire to maintain competitive advantage? And how can clinicians, the future users of these technologies, evaluate the risks for their patients and themselves amid such secrecy?
These are questions that Arti Rai, JD, and her team sought to explore with funding from a Making a Difference grant, Explainability and Trade Secrecy in AI-Enabled Clinical Decision Software.
“[AI] has established a substantial foothold in health care, including clinical decision-making, and has the potential to provide better clinical results less expensively,” Prof. Rai said in the Journal of Law and the Biosciences. However, she warns of potential pitfalls for relying on ML-CD for diagnoses, highlighting cases of inaccuracies that harm historically vulnerable groups.
Ethical issues can arise when using AI in medical settings due to unsuitable training and test data in the development stage. Whereas traditional rules-based software acts on data, ML-CD devices are built with data. Developers utilize learning algorithms to create software models that will translate inputs, such as a patient’s biometrics, into outputs such as recommended medications or treatments. The original dataset used to train and test ML-CD plays a key role in how the AI will ultimately function. In the Iowa Law Review, Prof. Rai and co-author W. Nicholson Price II, JD, PhD, assert that having “[i]nsight into the inputs influencing a model’s decision making can itself be important for ensuring reliable, repeated performance.” Thus, datasets used in development “should represent something close to ‘ground truth’.”
So, where along the way can trust in these systems break down? Prof. Rai and her team interviewed stakeholders and found that FDA clearance was a “helpful mark of quality and effectiveness,” but that the FDA is not always requesting detailed information about data and algorithms for products it reviews.
The researchers also learned that decision-makers and adopters alike are mainly interested in performance data. In particular, they are concerned about the relevance to their individual health system populations and therefore some prefer to test the ML-CD with their own data before full adoption.
“The Greenwall grant was critical for my team’s work. It provided important financial support for our quantitative and qualitative research, and it also gave us the benefit of the sterling reputation associated with the Greenwall name.”Arti Rai, JD
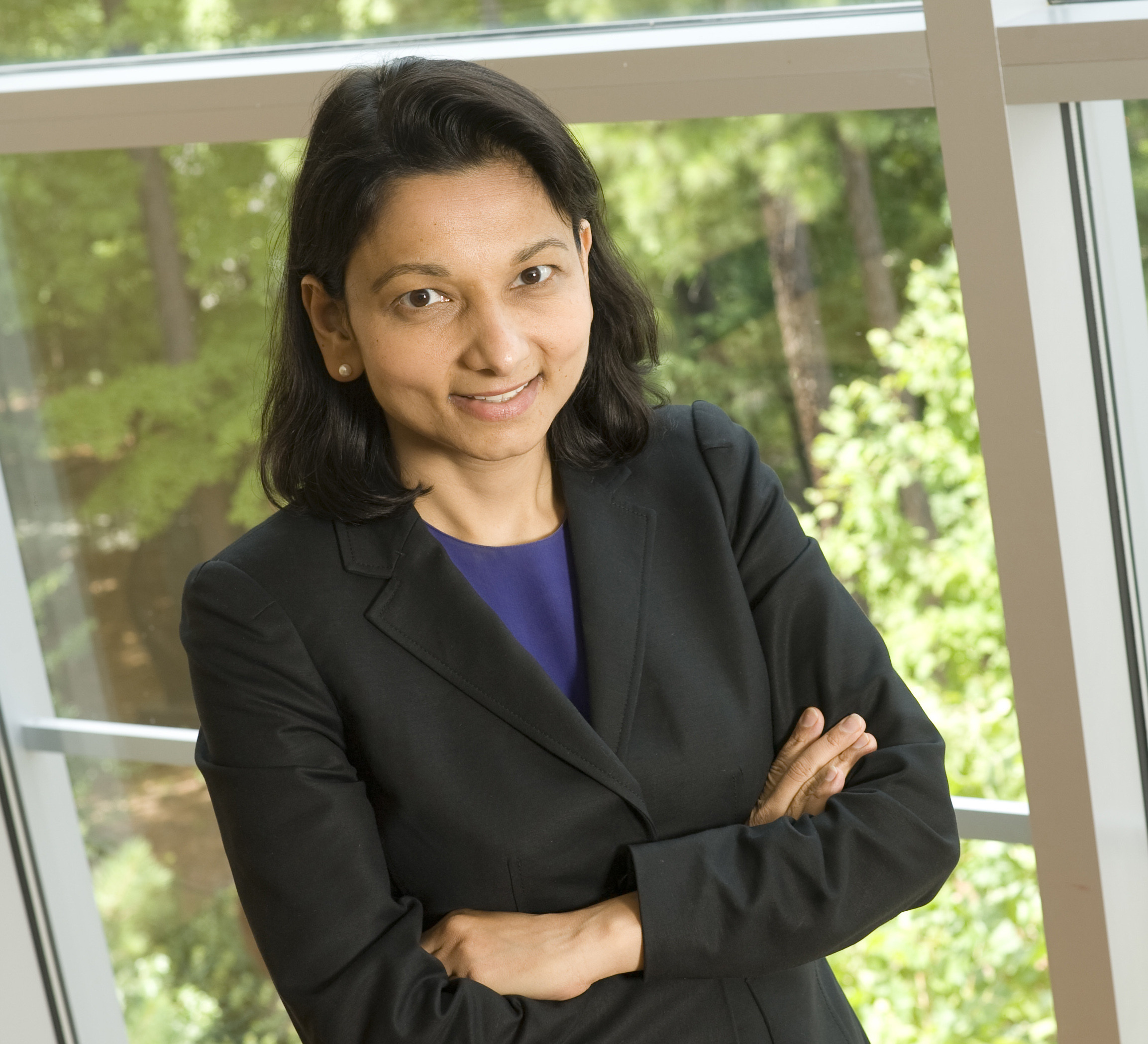
Finally, at the patient level, interviews showed mixed results. Patients were interested in AI to help with a diagnosis, but not provide the care directly. Overall, the researchers concluded that there needed to be adequate trust of the FDA’s ability to evaluate products rigorously while keeping training data and algorithms private; trust of developers to utilize appropriate training and test data; trust in health care systems to protect their clinicians from liability concerns; and trust in clinician end users to interpret AI outputs effectively.
Despite these hurdles, Prof. Rai does not want to detract from the benefits of AI in medical applications, a sentiment echoed by others in the medical sphere. She cites a survey from KMPG and analysis done by Accenture to conclude that AI can support greater access to care and address unmet clinical need in the years to come, underscoring the urgency in identifying and tackling challenges of ML-CD implementation.
In their published white paper, the research team laid out the following recommendations, among others:
- ML-CD manufacturers and health providers that use ML-CD should work together to curate a user experience that fits into health system workflows, and show evidence of the product’s clinical utility
- With higher risk AI products, trusted third parties (including but not limited to the FDA) should have procedures in place to securely evaluate “trade secret” information; additionally, stakeholders should have best practices for evaluating products before procurement and monitoring performance after implementation
- Summary information about AI training data, labeling methods, testing process, intended use, and input requirements should be disclosed publicly in a clear way
Prof. Rai is hopeful these recommendations will make a difference as ML-CD models begin to be used for riskier, but potentially highly beneficial, purposes: “The sum total of these pragmatic adjustments to existing regimes of information flow should move the needle on accountability without compromising innovation incentives.”